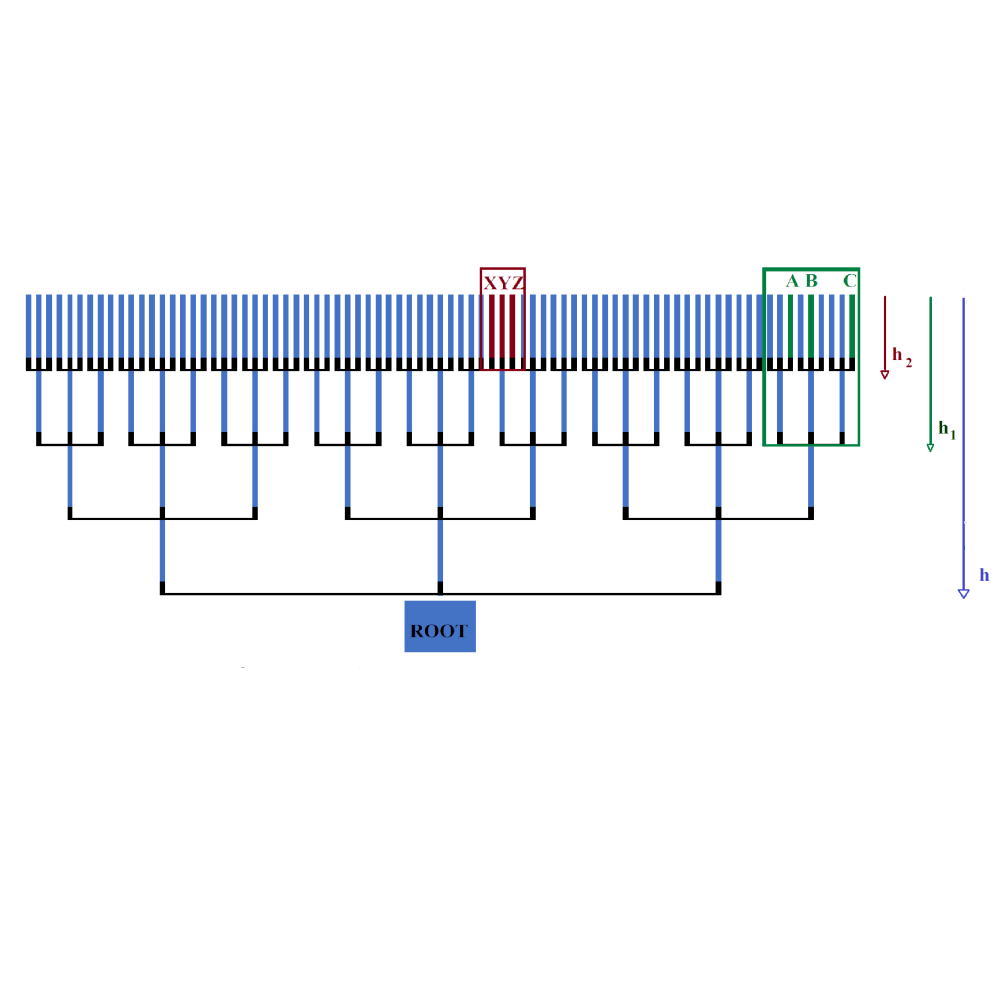
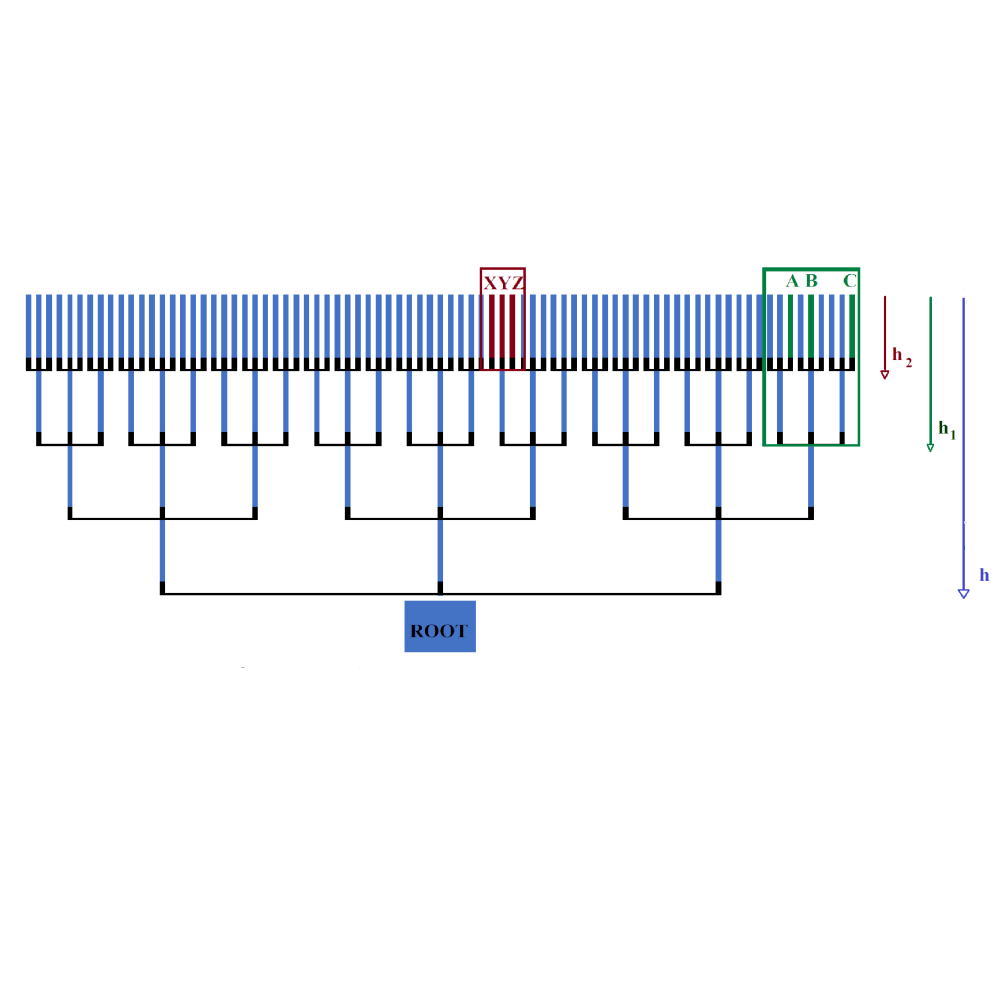
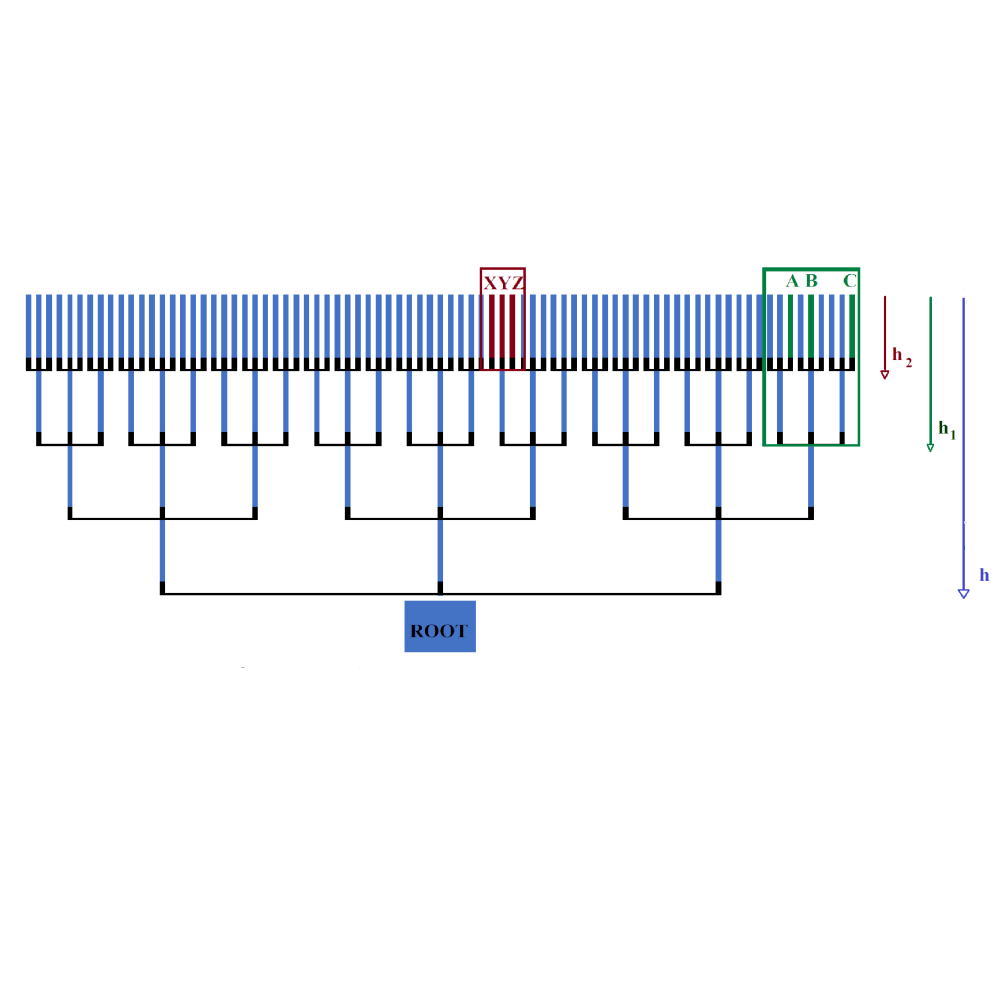
Determinantal point processes (DPPs) are popular tools that supply useful information for repulsiveness. They provide coherent probabilistic models when negative correlations arise and also represent new algorithms for inference problems like sampling, marginalization and conditioning. Recently, DPPs have played an increasingly important role in machine learning and statistics, since they are used for diverse subset selection problems. In this paper we use -DPP, a conditional DPP that models only sets of cardinality , to sample a diverse subset of species from a large phylogenetic tree. The tree sampling task is important in many studies in modern bioinformatics. The results show a fast mixing sampler for -DPP, for which a polynomial bound on the mixing time is given. This approach is applied to a real-world dataset of species, and we observe that leaves joined by a higher subtree are more likely to appear.
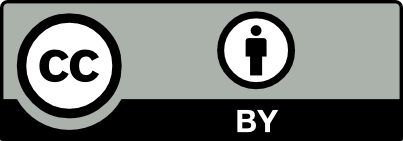
@article{MSIA_2021__10_1_1_0, author = {Diala Wehbe and Nicolas Wicker and Baydaa Al-Ayoubi and Luc Moulinier}, title = {Fixed-Size {Determinantal} {Point} {Processes} {Sampling} {For} {Species} {Phylogeny}}, journal = {MathematicS In Action}, pages = {1--13}, publisher = {Soci\'et\'e de Math\'ematiques Appliqu\'ees et Industrielles}, volume = {10}, number = {1}, year = {2021}, doi = {10.5802/msia.13}, language = {en}, url = {https://msia.centre-mersenne.org/articles/10.5802/msia.13/} }
TY - JOUR AU - Diala Wehbe AU - Nicolas Wicker AU - Baydaa Al-Ayoubi AU - Luc Moulinier TI - Fixed-Size Determinantal Point Processes Sampling For Species Phylogeny JO - MathematicS In Action PY - 2021 SP - 1 EP - 13 VL - 10 IS - 1 PB - Société de Mathématiques Appliquées et Industrielles UR - https://msia.centre-mersenne.org/articles/10.5802/msia.13/ DO - 10.5802/msia.13 LA - en ID - MSIA_2021__10_1_1_0 ER -
%0 Journal Article %A Diala Wehbe %A Nicolas Wicker %A Baydaa Al-Ayoubi %A Luc Moulinier %T Fixed-Size Determinantal Point Processes Sampling For Species Phylogeny %J MathematicS In Action %D 2021 %P 1-13 %V 10 %N 1 %I Société de Mathématiques Appliquées et Industrielles %U https://msia.centre-mersenne.org/articles/10.5802/msia.13/ %R 10.5802/msia.13 %G en %F MSIA_2021__10_1_1_0
Diala Wehbe; Nicolas Wicker; Baydaa Al-Ayoubi; Luc Moulinier. Fixed-Size Determinantal Point Processes Sampling For Species Phylogeny. MathematicS In Action, Tome 10 (2021) no. 1, pp. 1-13. doi : 10.5802/msia.13. https://msia.centre-mersenne.org/articles/10.5802/msia.13/
[1] Monte Carlo Markov chain algorithms for sampling strongly Rayleigh distributions and determinantal point processes, In COLT (2016)
[2] Negative dependence and the geometry of polynomials, J. Am. Math. Soc., Volume 22 (2009), pp. 521-567 | DOI | MR | Zbl
[3] On selecting a maximum volume sub-matrix of a matrix and related problems, Theor. Comput. Sci. (2009), pp. 4801-4811 | DOI | MR | Zbl
[4] Spectra of Graphs, Academic Press Inc., 1980 | Zbl
[5] Efficient volume sampling for row/column subset selection, FOCS (2010) no. 1-3-4, pp. 329-338
[6] Random Walks and Electric Networks, Mathematical Association of America, 1984 | Zbl
[7] Statistical ensembles of complex, quaternion,and real matrices, J. Math. Phys., Volume 6 (1965), pp. 440-449 | DOI | MR | Zbl
[8] Random walks on graphs., Stochastic Processes Appl., Volume 2 (1974), pp. 311-336 | DOI | MR | Zbl
[9] Fast determinantal point process sampling with application to clustering, NIPS (2013), pp. 2319-2327
[10] Spectral algorithms, Foundations and Trends in Theoretical Computer Science, Volume 4 (2009), pp. 157-288 | DOI | MR
[11] Diffusion kernels on graphs and other discrete structures, Proceedings of the 19th International Conference On Machine Learning (2002), pp. 315-322
[12] kDPPs: fixed size determinantal point processes, Proceedings of the 28th International Conference on Machine Learning, Omnipress, 2011, pp. 1193-1200
[13] Determinantal point processes for machine learning, Foundations and Trends in Machine Learning, Volume 5 (2012) no. 2-3, pp. 123-286 | DOI | Zbl
[14] Random walks on graphs: A survey, Combinatorics, Volume 2 (1993), pp. 1-46
[15] The Coincidence approach to stochastic point processes, Adv. Appl. Probab., Volume 7 (1975) no. 1, pp. 83-122 | DOI | MR | Zbl
[16] On the density of eigenvalues of a random matrix, Nuclear Physics, Volume 18 (1960), pp. 420-427 | DOI | MR | Zbl
[17] Laplacian matrices of graphs: A survey, Linear Algebra Appl., Volume 197-198 (1994), pp. 143-176 | DOI | MR | Zbl
[18] UniProt Protein Knowledgebase, Methods Mol. Biol, Volume 1558 (2017), pp. 41-55 | DOI
Cité par Sources :